Email Marketing Data Science
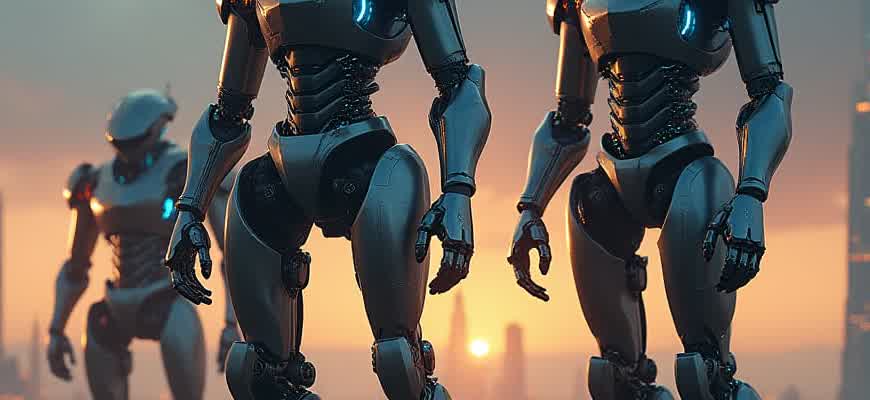
Email marketing has evolved into a data-driven practice, with data science playing a key role in optimizing campaign performance. By analyzing large datasets, businesses can make informed decisions that enhance the effectiveness of their email campaigns. The integration of data science techniques helps in identifying customer preferences, predicting behaviors, and personalizing content for increased engagement.
Below are the main areas where data science improves email marketing:
- Audience Segmentation: Data science enables marketers to divide their audience into more targeted groups based on behaviors, preferences, and demographics.
- Predictive Analytics: By using historical data, predictive models can estimate future user actions, helping businesses tailor email content accordingly.
- Performance Optimization: Continuous analysis of campaign results allows marketers to adjust subject lines, sending times, and content for optimal performance.
"Incorporating data science into email marketing allows companies to not only reach their audience but engage them in a meaningful way that drives conversions."
To demonstrate the impact of these strategies, consider the following table comparing traditional email marketing techniques versus data-driven approaches:
Technique | Traditional Approach | Data-Driven Approach |
---|---|---|
Segmentation | Basic demographic categories | Advanced behavior-based segmentation |
Content Personalization | Generic content for all users | Dynamic content tailored to individual preferences |
Optimization | Manual A/B testing | Automated performance tracking and adjustments |
Optimizing Email Open Rates with Predictive Analytics
Email marketing effectiveness heavily relies on engagement metrics, with open rate being one of the most critical indicators. Understanding factors influencing this metric can guide marketers in refining their strategies. Predictive modeling offers a sophisticated approach to forecast open rates, helping businesses optimize email campaigns by targeting the right audience at the right time with the most relevant content.
By leveraging machine learning algorithms, data scientists can analyze past interactions and user behavior to predict the likelihood of an email being opened. This method takes into account numerous factors such as subject line effectiveness, send time, sender reputation, and recipient engagement history. Here’s how predictive models can transform email marketing strategies:
Key Steps in Predictive Analysis for Open Rates
- Data Collection: Gather historical email performance data, including open rates, click-through rates, and user demographics.
- Feature Engineering: Identify key features influencing open rates, such as subject line length, time of day, and previous engagement patterns.
- Model Selection: Choose an appropriate machine learning model (e.g., logistic regression, random forest, or neural networks) for prediction.
- Model Evaluation: Assess model accuracy using metrics like precision, recall, and F1 score to ensure predictive reliability.
Effective predictive modeling allows marketers to anticipate user behavior and adjust their strategies proactively, leading to higher engagement rates.
Example of a Predictive Model in Action
Feature | Impact on Open Rate |
---|---|
Subject Line Length | Shorter subject lines tend to increase open rates by 15% |
Send Time | Emails sent on weekdays between 10 AM and 2 PM see higher open rates |
Personalization | Personalized subject lines improve open rates by 20% |
By applying predictive analytics, marketers can not only forecast open rates but also identify actionable insights to enhance future campaigns. The process of refining these models and constantly testing new hypotheses is crucial for staying ahead in a competitive landscape.
Optimizing Email Campaigns Through Customer Segmentation
Customer segmentation is a fundamental strategy in email marketing that allows businesses to tailor their messaging and content more effectively to different groups within their audience. By dividing customers into smaller, more specific segments, brands can increase the relevance of their communication, driving higher engagement and conversion rates. Advanced data science techniques provide valuable insights that enable marketers to create targeted campaigns that resonate with distinct customer needs and behaviors.
Leveraging this segmentation not only improves the effectiveness of campaigns but also enhances customer loyalty and retention. Through the strategic use of segmented data, email marketing efforts can be aligned with the specific preferences, purchasing habits, and demographic characteristics of individual users. This ensures that each customer receives the most appropriate content, offers, and messaging at the right time.
Key Benefits of Customer Segmentation in Email Campaigns
- Personalized Messaging: Tailor content to individual preferences and behaviors, creating a more personalized experience.
- Improved Engagement: Sending relevant offers increases open rates, click-through rates, and conversions.
- Enhanced Customer Loyalty: Customers are more likely to engage with brands that understand their needs and deliver appropriate messaging.
Effective Segmentation Strategies
- Behavioral Segmentation: Group customers based on their previous interactions with emails, website visits, and purchase history.
- Demographic Segmentation: Use data like age, gender, location, or income to create personalized messages tailored to specific customer groups.
- Engagement Segmentation: Categorize customers based on their level of engagement, distinguishing between highly engaged users and those with less interaction.
Example of a Customer Segmentation Table
Segment | Characteristics | Preferred Content | Message Type |
---|---|---|---|
Frequent Shoppers | High purchase frequency, loyal to brand | Exclusive offers, loyalty rewards | Promotional, personalized discounts |
New Customers | First-time purchasers or subscribers | Welcome emails, product recommendations | Informational, onboarding series |
Inactive Customers | Low interaction, no purchases in last 3 months | Re-engagement offers, reminders | Win-back, time-sensitive promotions |
Tip: Continuous monitoring and updating of customer segments are essential to ensure email campaigns stay relevant and impactful.
Optimizing Email Delivery Times Using Data-Driven Approaches
Choosing the right time to send an email is crucial for maximizing engagement and conversion rates. By leveraging historical data and analyzing user behavior, businesses can tailor their email campaigns to specific timeframes that are most likely to yield positive results. This approach moves beyond generic best practices and ensures that messages reach subscribers when they are most likely to open and engage with them.
Data analysis can reveal patterns related to the best send times for different customer segments. Factors like time zone, user activity, and past email interactions are important considerations that can help improve email performance. By applying data-driven insights, marketers can enhance open rates, click-through rates (CTR), and overall campaign success.
Factors Affecting Optimal Email Send Times
- Time Zone Variations: Consider the geographic location of your subscribers to send emails during their peak hours.
- User Behavior: Identify when your audience is most active, using previous interactions to determine patterns.
- Day of the Week: Some days of the week show higher engagement rates depending on your audience’s habits.
Methods for Data-Driven Time Optimization
- Analyze historical email performance data.
- Segment audiences based on their interaction patterns.
- Test different send times for A/B testing.
- Use machine learning models to predict optimal delivery windows.
Data insights from previous campaigns can provide powerful signals about when your subscribers are most likely to engage with emails, offering a clear path to improved outcomes.
Example of Performance Analysis
Day | Open Rate | Click-through Rate |
---|---|---|
Monday | 18% | 3.5% |
Wednesday | 22% | 4.1% |
Friday | 20% | 3.8% |
Refining Email Campaigns with A/B Testing Insights
In email marketing, optimizing subject lines and content is crucial for maximizing engagement and conversion rates. A/B testing provides valuable data on how slight modifications can impact recipient behavior. By testing different versions of subject lines or content formats, marketers can identify the most effective approaches for specific segments of their audience. This method allows for evidence-based decisions rather than relying on assumptions, enhancing the overall effectiveness of email campaigns.
Utilizing A/B test results, marketers can continuously improve their emails. Analyzing the data from tests helps refine messaging strategies, ensuring that emails resonate with recipients, resulting in higher open rates, click-through rates, and, ultimately, better campaign performance. Below are some practical ways to leverage A/B testing data for refining subject lines and email content.
Optimizing Subject Lines with A/B Testing
Subject lines are one of the first things recipients see, and they significantly influence whether an email is opened. A/B testing allows for testing different elements of a subject line to understand what drives engagement. Some key areas to test include:
- Personalization – Experiment with using the recipient's name or other personalized data.
- Length – Test short vs. longer subject lines to see which performs better.
- Urgency – Analyze the impact of time-sensitive language on open rates.
- Tone – Test whether a formal or informal tone is more effective for your audience.
Testing subject lines should be an ongoing process. Trends shift over time, and what works now might not work as well in the future.
Improving Email Content Based on A/B Test Data
Refining the content within the email itself is just as important as optimizing the subject line. A/B testing content elements, such as the placement of calls to action (CTAs), visual design, and copy, can significantly affect how recipients engage with the email.
- CTA Placement – Test whether placing a CTA above or below the fold increases click-through rates.
- Copy Tone – Evaluate whether a casual tone or a more professional approach results in better engagement.
- Visual Appeal – Experiment with different image styles and sizes to see which drives better results.
Test Results: Key Metrics to Track
To understand the effectiveness of your tests, it’s crucial to track key performance indicators (KPIs) throughout the campaign. Below is a table of essential metrics to monitor:
Metric | What to Measure |
---|---|
Open Rate | Percentage of recipients who opened the email |
Click-through Rate (CTR) | Percentage of recipients who clicked on a link within the email |
Conversion Rate | Percentage of recipients who completed the desired action (purchase, sign-up, etc.) |
Unsubscribe Rate | Percentage of recipients who unsubscribed after receiving the email |
Regularly monitoring these KPIs ensures you can effectively compare the impact of different subject lines and content variations.
Integrating Customer Lifetime Value (CLV) Metrics into Campaign Strategy
Understanding the long-term value of a customer is crucial in optimizing email marketing efforts. By integrating customer lifetime value (CLV) metrics, marketers can design campaigns that target high-value individuals while improving overall return on investment (ROI). CLV provides insights into how much revenue a customer is expected to generate during their relationship with the brand, allowing businesses to prioritize efforts towards retaining these high-value segments.
To successfully integrate CLV into email marketing, data scientists and marketers must first define the right model for predicting customer value. Once this model is in place, the next step is to tailor campaigns accordingly, ensuring that the most valuable customers are nurtured while optimizing resources for other segments based on their predicted CLV.
Key Actions for CLV Integration
- Segment Customers based on their predicted CLV to create targeted email lists.
- Prioritize High-Value Segments with personalized offers, loyalty programs, and exclusive content.
- Utilize CLV for Content Personalization by tailoring email messages and offers to reflect individual customer behaviors and preferences.
- Optimize Frequency and Timing of emails for different customer segments to avoid over-saturation while maintaining engagement.
CLV-Based Campaign Example
- Identify High CLV Segments: Use data analytics to categorize customers by their lifetime value.
- Personalized Campaigns: Send specific promotions based on the predicted needs or interests of these segments.
- Measure Impact: Track engagement and revenue generated from high-value segments to adjust future strategies.
- Iterate and Refine: Continuously update CLV predictions and adjust campaigns for improved performance.
Important Considerations
Integrating CLV into your email marketing strategy requires constant refinement. As customer behavior evolves, it is essential to update your CLV models regularly to ensure accurate predictions and relevant messaging.
CLV Table Example
Customer Segment | Average CLV | Campaign Focus |
---|---|---|
High CLV | $500+ | Exclusive promotions, VIP offers, loyalty rewards |
Medium CLV | $200 - $500 | Regular discounts, product recommendations |
Low CLV | Under $200 | Engagement content, incentives to increase purchases |
Minimizing Unsubscribe Rates through Risk Factor Analysis
Email marketing campaigns are a crucial tool for engaging with customers and driving conversions. However, a high unsubscribe rate can signal that your audience is disengaging with your content. Reducing these rates involves understanding and addressing the key factors that contribute to this behavior. Data science offers powerful methods for identifying patterns that lead to unsubscribes, allowing marketers to take proactive measures to retain subscribers.
To minimize unsubscribe rates, it’s important to focus on several key risk factors that can indicate when a subscriber is likely to opt-out. By leveraging historical campaign data and advanced analytics, marketers can identify these risk factors early on. These insights allow for targeted strategies aimed at improving content relevance, frequency, and personalization, ultimately reducing churn.
Key Risk Factors for Unsubscribing
- Excessive Email Frequency: Sending emails too frequently can overwhelm subscribers, leading to frustration and opt-outs.
- Lack of Personalization: Generic email content that doesn't address individual preferences or behavior can result in disinterest and unsubscribes.
- Unclear Value Proposition: When subscribers fail to see the benefits of staying subscribed, they are more likely to disengage.
- Irrelevant Content: Emails that are not aligned with a subscriber’s interests or needs tend to drive them away.
- Inconsistent Branding: Emails that lack a consistent voice or design can confuse subscribers, prompting them to unsubscribe.
Approach to Reducing Unsubscribe Risk
- Segment Your Audience: Use data analysis to group subscribers based on their behavior, interests, and past interactions. Tailor content to these segments to ensure relevance.
- Monitor Engagement Metrics: Track open rates, click-through rates, and unsubscribes to spot patterns that indicate potential issues.
- Optimize Email Frequency: Use predictive models to find the optimal sending frequency for different segments, avoiding over-saturation.
- Personalize Content: Leverage dynamic content generation to ensure that emails speak directly to the needs and preferences of each subscriber.
- A/B Testing: Regularly test different subject lines, content formats, and sending times to identify what resonates best with your audience.
"By identifying high-risk subscribers and taking proactive action, marketers can significantly reduce unsubscribe rates and improve the overall effectiveness of their campaigns."
Example of Risk Factor Analysis
Risk Factor | Impact on Unsubscribe Rate | Action to Mitigate |
---|---|---|
High Email Frequency | Increases the likelihood of opt-outs due to over-saturation | Implement frequency caps and personalize sending schedules |
Lack of Personalization | Can result in irrelevant content and disengagement | Use dynamic content and behavioral data to personalize emails |
Irrelevant Content | Leads to decreased interest and higher unsubscribe rates | Segment audience and tailor content to their preferences |
How Machine Learning Enhances the Prediction of Email Campaign Click-Through Rates
Machine learning has become a crucial tool in optimizing email marketing campaigns by predicting the likelihood of recipients clicking on links within an email. By analyzing historical data, ML models can detect patterns in user behavior and adjust the content and timing of emails to maximize engagement. This allows marketers to tailor their campaigns more effectively and make data-driven decisions. The use of algorithms helps refine targeting strategies, providing a more personalized experience for each recipient.
Through the use of supervised learning techniques, algorithms can predict the probability of a click-through based on a variety of factors, including demographic information, past interactions, and the content of the email. These predictions can then guide the creation of future campaigns by identifying elements that are most likely to engage subscribers. As the model learns over time, its accuracy improves, leading to increasingly effective email marketing strategies.
Factors Influencing Click-Through Predictions
- User Behavior: Previous engagement with emails, such as open rates and past clicks, is analyzed to predict future actions.
- Email Content: Subject lines, design, and CTA (Call-to-Action) buttons are evaluated for their impact on user engagement.
- Time and Frequency: The optimal time and frequency of sending emails are determined based on user activity patterns.
- Demographics: Age, location, and other demographic data play a role in predicting response rates.
Machine Learning Process for Predicting Click-Through Rates
- Data Collection: Historical email campaign data is collected, including open rates, click-through rates, time of interaction, and user demographics.
- Feature Engineering: Relevant features are extracted from raw data, such as subject line length, email type, and past behaviors.
- Model Training: A machine learning model is trained on the data using algorithms like logistic regression, decision trees, or neural networks.
- Prediction: The trained model is used to predict future click-through rates based on new email campaign parameters.
- Optimization: The model's output is analyzed to optimize future campaign strategies for higher engagement.
Example Model Prediction Accuracy
Model Type | Training Accuracy | Prediction Accuracy |
---|---|---|
Logistic Regression | 85% | 80% |
Decision Tree | 90% | 87% |
Neural Networks | 95% | 92% |
Key Insight: Machine learning's ability to analyze large datasets and identify patterns is critical for predicting email campaign success. Over time, continuous training and model refinement can lead to even more accurate predictions, helping marketers fine-tune their approach and improve overall campaign performance.